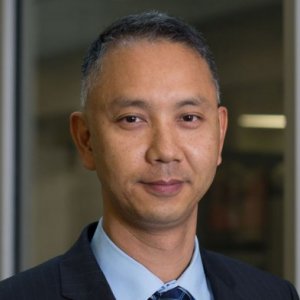
Yuichi Motai, Ph.D.
Associate Professor, Department of Electrical and Computer Engineering
- Engineering West Hall, Room 212, Richmond VA UNITED STATES
Associate Professor of the Department of Electrical and Computer Engineering of Virginia Commonwealth University.
Links
Social
Biography
Industry Expertise
Areas of Expertise
Education
Purdue University
Ph.D.
Electrical and Computer Engineering
2002
Kyoto University
M.E.
Applied Systems Science
1993
Keio University
B.E.
Instrumentation Engineering
1991
Affiliations
- Virginia Commonwealth University
- Sigma Xi (Scientific Research Society)
- IEEE (Institute of Electrical and Electronics Engineers) Senior member
- ACM (Association for Computing Machinery) Lifetime member
- ASEE (American Society for Engineering Education)
Media Appearances
Editor's Note
IEEE Intelligent transportation systems magazine print
2017-07-25
Review of the Book Predicting Vehicle Trajectory [Book Review] Christos-Nikolaos E. Anagnostopoulos
IEEE Intelligent Transportation Systems Magazine
Year: 2017, Volume: 9, Issue: 3
Pages: 156 - 157, DOI: 10.1109/MITS.2017.2711255
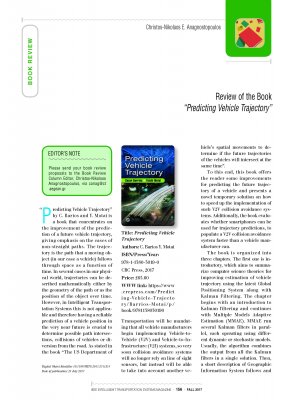
Research Grants
CAREER: Engineering Data-intensive Prediction and Classification for Medical Testbeds with Nonlinear, Distributed, and Interdisciplinary Approaches
NSF $448000
2011-02-01
Research Objectives and Approaches
The objective of this research is to contribute to the interdisciplinary topic on STEM and basic medical science, specifically Patient-Centered Health Informatics Applications, with the newly proposed techniques that stand to benefit from the investigator?s expertise in engineering and from the collaboration with medical experts.
The approach is to study the medical data from several institutions comprehensively with the dynamics of all the datasets in their entirety such as non-linearity with kernel factors, and the network characteristics of whole multiple database distribution, rather than applying traditional techniques of prediction and classification to the very limited number of small medical testbeds.
Intellectual Merit
When the proposed adaptive tracking method is used on soft tissue tumors, radiosurgery systems maintain precise targeting of the tumor by predicting tumor motion using a motion tracking system. The successful development of the proposed dynamic classification method will substantially advance the clinical implementation of cancer screening, promote the early diagnosis of colon cancer, lead to an improved screening rate, and ultimately contribute toward reducing the mortality due to colon cancer.
Broader impacts
The proposed data-intensive solutions can save millions of cancer patients every year. The expected outcomes will be applied to medical problems and benefit society as a whole by enhancing the quality of all our lives, through unprecedented advances in the early diagnosis and treatment of cancer. The advancements in the developed framework will make use of and expand the Nation's cyber infrastructure and high performance computing capability.
CRADA ARL JWS 15-18-01 Omni-Directional Infrared Imagery to Enhance Localization and Tracking Algorithms
U.S. ARMY Research Laboratory $30163
2015-09-01
Robot Vision
NIH R01 CA159471-07 Subaward – Washington University
National Institutes of Health $25099
2015-12-01
Medical Imaging
Courses
Signals and Systems I
Presents the concept of linear continuous-time and discrete-time signals and systems, their classification, and analysis and design using mathematical models. Topics to be covered: the concepts of linear systems and classification of these systems, continuous-time linear systems and differential and difference equations, convolution, frequency domain analysis of systems, Fourier series and Fourier transforms and their application, and continuous-time to discrete-time conversion, and Laplace transformation and Transfer function representation.
Dynamic and Multivariable Systems
This course covers the use of state space methods to model analog and digital linear and nonlinear systems. Emphasis is placed on the student gaining mathematical modeling experience, performing sensitivity and stability analysis and designing compensators to meet systems specifications. Topics treated will include a review of root locus and frequency design methods, linear algebraic equations, state variable equations, state space design and digital control systems (principles and case studies). The students will use complex dynamic systems for analysis and design. The laboratory will consist of modeling and control demonstrations and experiments single-input/single-output and multivariable systems, analysis and simulation using matlab control toolbox and other control software.
Automatic Control
This course covers the design and analysis of linear feedback systems. Emphasis is placed upon the student gaining mathematical modeling experience and performing sensitivity and stability analysis. The use of compensators to meet systems design specifications will be treated. Topics include: an overview and brief history of feedback control, dynamic models, dynamic response, basic properties of feedback, root-locus, frequency response and state space design methods. The laboratory will consist of modeling and control demonstrations and experiments single-input/single-output and multivariable systems, analysis and simulation using matlab/simulink and other control system analysis/design/implementation software.
Pattern Recognition
This course will give an introduction to statistical pattern classification. The fundamental background for the course is probability theory, especially those fundamental topics summarized in Appendix of the text. The course is suitable for students, in engineering, mathematics, and computer science, who have a basic background in calculus, linear algebra, and probability theory, and who have some interest in exploring the field of pattern recognition. The course will closely follow the material in the text, and will be surveyed through the most chapters 1-10 (except 7). The intention is to spend about 3 class periods discussing material selectively covered in each chapter. Students are expected to read each chapter, prior to the start of the second class on that chapter.
Digital Signal Processing
The course focuses on digital signal processing theory and algorithms, including sampling theorems, transform analysis and filter design techniques. Discrete-time signals and systems, and filter design techniques are treated. Several applications of DSP in telecommunications, image and video processing, and speech and audio processing are studied.
Selected Articles
Heterogeneous data analysis: Online learning for medical-image-based diagnosis
Pattern Recognition2017-01-01
Heterogeneous Data Analysis (HDA) is proposed to address a learning problem of medical image databases of Computed Tomographic Colonography (CTC). The databases are generated from clinical CTC images using a Computer-aided Detection (CAD) system, the goal of which is to aid radiologists' interpretation of CTC images by providing highly accurate, machine-based detection of colonic polyps. We aim to achieve a high detection accuracy in CAD in a clinically realistic context, in which additional CTC cases of new patients are added regularly to an existing database. In this context, the CAD performance can be improved by exploiting the heterogeneity information that is brought into the database through the addition of diverse and disparate patient populations. In the HDA, several quantitative criteria of data compatibility are proposed for efficient management of these online images. After an initial supervised offline learning phase, the proposed online learning method decides whether the online data are heterogeneous or homogeneous. Our previously developed Principal Composite Kernel Feature Analysis (PC-KFA) is applied to the online data, managed with HDA, for iterative construction of a linear subspace of a high-dimensional feature space by maximizing the variance of the non-linearly transformed samples. The experimental results showed that significant improvements in the data compatibility were obtained when the online PC-KFA was used, based on an accuracy measure for long-term sequential online datasets. The computational time is reduced by more than 93% in online training compared with that of offline training.
Intra- and Inter-Fractional Variation Prediction of Lung Tumors Using Fuzzy Deep Learning
IEEE Journal of Translational Engineering in Health and Medicine2015-12-01
Tumor movements should be accurately predicted to improve delivery accuracy and reduce unnecessary radiation exposure to healthy tissue during radiotherapy. The tumor movements pertaining to respiration are divided into intra-fractional variation occurring in a single treatment session and inter-fractional variation arising between different sessions. Most studies of patients' respiration movements deal with intra-fractional variation. Previous studies on inter-fractional variation are hardly mathematized and cannot predict movements well due to inconstant variation. Moreover, the computation time of the prediction should be reduced. To overcome these limitations, we propose a new predictor for intra- and inter-fractional data variation, called intra- and inter-fraction fuzzy deep learning (IIFDL), where FDL, equipped with breathing clustering, predicts the movement accurately and decreases the computation time. Through the experimental results, we validated that the IIFDL improved root-mean-square error (RMSE) by 29.98% and prediction overshoot by 70.93%, compared with existing methods. The results also showed that the IIFDL enhanced the average RMSE and overshoot by 59.73% and 83.27%, respectively. In addition, the average computation time of IIFDL was 1.54 ms for both intra- and inter-fractional variation, which was much smaller than the existing methods. Therefore, the proposed IIFDL might achieve real-time estimation as well as better tracking techniques in radiotherapy.
Trajectory Estimations Using Smartphones
IEEE Transactions on Industrial Electronics2015-06-01
This paper investigates whether the smartphones' built-in sensors can accurately predict future trajectories for a possible implementation in a vehicle-to-vehicle (V2V) and vehicle-to-infrastructure (V2I) system. If smartphones could be used, vehicles without the V2V/V2I technology could use them to tap into the V2V/V2I infrastructure and help to populate the gap of vehicles off the V2V/V2I grid. To evaluate this, we set up a dead-reckoning system that uses Kalman filters to predict the future trajectory of a vehicle, information that could be used in a V2V/V2I system to warn drivers if the trajectories of vehicles will intersect at the same time. Then, we use a vehicle with accelerometer, GPS, and speedometer sensors mounted on it and evaluate its accuracy in predicting the future trajectory. Afterward, we place a smartphone securely on the vehicle's dashboard, and we use its internal accelerometer and GPS to feed the same dead reckoning and Kalman filter setup to predict the future trajectory of the vehicle. We end by comparing both results and evaluating if a smartphone can achieve similar accuracy in predicting the future trajectory of a vehicle. Our results show that some smartphones could be used to predict a future position, but the use of their accelerometer sensors introduces some measurements that can be incorrectly interpreted as spatial changes.
Intelligent Forecasting using Dead Reckoning with Dynamic Errors
IEEE Transactions on Industrial Informatics2016-06-01
A method for integrating, processing, and analyzing sensing data from vehicle-mounted sensors for intelligent forecasting and decision-making is introduced. This dead reckoning with dynamic errors (DRWDEs) is for a large-scale integration of distributed resources and sensing data intervehicle collision avoidance system. This sensor fusion algorithm is introduced to predict the future trajectory of a vehicle. Current systems that predict a vehicle’s future trajectory, necessary in a network of collision avoidance systems, tend to have a lot of errors when the vehicles are moving in a nonstraight path. Our system has been designed with the objective of improving the estimations during curves. To evaluate this system, our research uses a Garmin 16HVS GPS sensor, an AutoEnginuity OBDII ScanTool, and a Crossbow three-axis accelerometer. Using Kalman filters (KFs), a dynamic noise covariance matrix merged together with an interacting multiple models (IMMs) system, our DRWDE produces the future position estimation of where the vehicle will be 3 s later in time. The ability to handle the change in noise, depending on unavailable sensor measurements, permits a flexibility to use any type of sensor and still have the system run at the fastest frequency available. Compared with a more common KF implementation that runs at the rate of its slowest sensor (1 Hz in our setup), our experimental results showed that our DRWDE (running at 10 Hz) yielded more accurate predictions (25%–50% improvement) during abrupt changes in the heading of the vehicle.
Smart Anomaly Prediction in Non-stationary CT Colonography Screening
IEEE Transactions on Industrial Informatics2016-06-01
To enhance the quality of economically efficient healthcare, we propose a preventive planning service for next-generation screening based on a longitudinal prediction. This newly proposed framework may bring important advancements in prevention by identifying the early stages of cancer, which will help in further diagnoses and initial treatment planning. The preventive service may also solve the obstacles of cost and availability of scanners in screening. For nonstationary medical data, anomaly detection is the key problem in the prediction of cancer staging. To address anomaly detection in a huge stream of databases, we applied a composite kernel to the prediction of cancer staging for the first time. The proposed longitudinal analysis of composite kernels (LACK) is designed for the prediction of anomaly status and cancer stage for further diagnosis and the future likelihood of cancer stage progression. The prediction error of LACK is relatively small even if the prediction is made far ahead of time. The computation time for nonstationary learning is reduced by 33% compared with stationary learning.
Cloud Colonography: Distributed Medical Testbed over Cloud
IEEE Transactions on Cloud Computing2016-06-01
Cloud Colonography is proposed in this paper, using different types of cloud computing environments. The sizes of the databases from the Computed Tomographic Colonography (CTC) screening tests among several hospitals are explored. These networked databases are going to be available in the near future via cloud computing technologies. Associated Multiple Databases (AMD) was developed in this study to handle multiple CTC databases. When AMD is used for assembling databases, it can achieve very high classification accuracy. The proposed AMD has the potential to play as a core classifier tool in the cloud computing framework. AMD for multiple institutions databases yields high detection performance of polyps using Kernel principal component analysis (KPCA). Two cases in the proposed cloud platform are private and public. We adapted a University cluster as a private platform, and Amazon Elastic Compute Cloud (EC2) as a public. The computation time, memory usage, and running costs were compared using three respresentative databases between private and public cloud environments. The proposed parallel processing modules improved the computation time, especially for the public cloud enviroments. The successful development of a cloud computing environment that handles large amounts of data will make Cloud Colonography feasible for a new health care service.
Dynamic 3D Surface Reconstruction and Motion Modeling from a Pan-Tilt-Zoom Camera
International Journal of Computers in Industries2015-06-01
3D surface reconstruction and motion modeling has been integrated in several industrial applications. Using a pan–tilt–zoom (PTZ) camera, we present an efficient method called dynamic 3D reconstruction (D3DR) for recovering the 3D motion and structure of a freely moving target. The proposed method estimates the PTZ measurements to keep the target in the center of the field of view (FoV) of the camera with the same size. Feature extraction and tracking approach are used in the imaging framework to estimate the target's translation, position, and distance. A selection strategy is used to select keyframes that show significant changes in target movement and directly update the recovered 3D information. The proposed D3DR method is designed to work in a real-time environment, not requiring all frames captured to be used to update the recovered 3D motion and structure of the target. Using fewer frames minimizes the time and space complexity required. Experimental results conducted on real-time video streams using different targets to prove the efficiency of the proposed method. The proposed D3DR has been compared to existing offline and online 3D reconstruction methods, showing that it uses less execution time than the offline method and uses an average of 49.6% of the total number of frames captured.
Smart Colonography Computing for Distributed Medical Databases with Group Kernel Feature Analysis
ACM Transactions on Intelligent Systems and Technologies2015-04-01
Computer-Aided Detection (CAD) of polyps in Computed Tomographic (CT) colonography is currently very limited since a single database at each hospital/institution doesn't provide sufficient data for training the CAD system's classification algorithm. To address this limitation, we propose to use multiple databases, (e.g., big data studies) to create multiple institution-wide databases using distributed computing technologies, which we call smart colonography. Smart colonography may be built by a larger colonography database networked through the participation of multiple institutions via distributed computing. The motivation herein is to create a distributed database that increases the detection accuracy of CAD diagnosis by covering many true-positive cases. Colonography data analysis is mutually accessible to increase the availability of resources so that the knowledge of radiologists is enhanced. In this article, we propose a scalable and efficient algorithm called Group Kernel Feature Analysis (GKFA), which can be applied to multiple cancer databases so that the overall performance of CAD is improved. The key idea behind the proposed GKFA method is to allow the feature space to be updated as the training proceeds with more data being fed from other institutions into the algorithm. Experimental results show that GKFA achieves very good classification accuracy.
Kernel Association for Classification and Prediction: a Survey
IEEE Transactions on Neural Networks and Learning Systems2015-02-01
Kernel association (KA) in statistical pattern recognition used for classification and prediction have recently emerged in a machine learning and signal processing context. This survey outlines the latest trends and innovations of a kernel framework for big data analysis. KA topics include offline learning, distributed database, online learning, and its prediction. The structural presentation and the comprehensive list of references are geared to provide a useful overview of this evolving field for both specialists and relevant scholars.
Scale-Invariant Tracking Optical Flow in Tracking using a Pan-Tilt-Zoom Camera
Robotica2015-04-01
An efficient method for tracking a target using a single Pan-Tilt-Zoom (PTZ) camera is proposed. The proposed Scale-Invariant Optical Flow (SIOF) method estimates the motion of the target and rotates the camera accordingly to keep the target at the center of the image. Also, SIOF estimates the scale of the target and changes the focal length relatively to adjust the Field of View (FoV) and keep the target appear in the same size in all captured frames. SIOF is a feature-based tracking method. Feature points used are extracted and tracked using Optical Flow (OF) and Scale-Invariant Feature Transform (SIFT). They are combined in groups and used to achieve robust tracking. The feature points in these groups are used within a twist model to recover the 3D free motion of the target. The merits of this proposed method are (i) building an efficient scale-invariant tracking method that tracks the target and keep it in the FoV of the camera with the same size, and (ii) using tracking with prediction and correction to speed up the PTZ control and achieve smooth camera control. Experimental results were performed on online video streams and validated the efficiency of the proposed method SIOF, comparing with OF, SIFT, and other tracking methods. The proposed SIOF has around 36% less average tracking error and around 70% less tracking overshoot than OF.
Design of a Fiber-optic Sensing Mosquito Trap
IEEE Sensors Journal2013-11-01
Mosquito traps offer researchers and health officials a reasonable estimate of mosquito abundances to assess the spatial and temporal occurrences of mosquito-transmitted pathogens. Existing traps, however, have issued efficient design to detect mosquito and energy consumption of the device. We designed a novel mosquito collection device that sensitively detects the presence of a mosquito via a fiber-optic sensor. In this prototype, a pushing capture mechanism selectively powers and efficiently captures live mosquitoes without destroying identifying morphological features of the specimens. Because the trap sensor selectively powers the capture mechanism, it allows for greatly reduced power consumption when compared with existing continuously operated devices. With appropriate programming, the fans ON and OFF based on the triggering of a fiber-optic sensor detected and counted each mosquito that entered the trap. This trapping platform can be used with a variety of power sources including renewable sources (e.g., solar, wind, or hydroelectric power) in remote settings. The experimental results show a high success ratio 93%-100% for detection of live mosquitoes.
Tracking Human Motion With Multichannel Interacting Multiple Model
IEEE Transactions on Industrial Informatics2013-03-01
Tracking human motion with multiple body sensors has the potential to promote a large number of applications such as detecting patient motion, and monitoring for home-based applications. With multiple sensors, the tracking system architecture and data processing cannot perform the expected outcomes because of the limitations of data association. For the collaborative and intelligent applications of motion tracking (Polhemus Liberty AC magnetic tracker), we propose a human motion tracking system with multichannel interacting multiple model estimator (MC-IMME). To figure out interactive relationships among distributed sensors, we used a Gaussian mixture model (GMM) for clustering. With a collaborative grouping method based on GMM and expectation-maximization algorithm for distributed sensors, we can estimate the interactive relationship with multiple body sensors and achieve the efficient target estimation to employ a tracking relationship within a cluster. Using multiple models with filter divergence, the proposed MC-IMME can achieve the efficient estimation of the measurement and the velocity from measured datasets of human sensory data. We have newly developed MC-IMME to improve overall performance with a Markov switch probability and a proper grouping method. The experiment results shows that the prediction overshoot error can be improved on average by 19.31% by employing a tracking relationship.
Local Intensity Feature Tracking and Motion Modeling for Respiratory Signal Extraction in Cone Beam CT Projections
IEEE Transactions on Biomedical Engineering2012-06-01
Accounting for respiration motion during imaging can help improve targeting precision in radiation therapy. We propose local intensity feature tracking (LIFT), a novel markerless breath phase sorting method in cone beam computed tomography (CBCT) scan images. The contributions of this study are twofold. First, LIFT extracts the respiratory signal from the CBCT projections of the thorax depending only on tissue feature points that exhibit respiration. Second, the extracted respiratory signal is shown to correlate with standard respiration signals. LIFT extracts feature points in the first CBCT projection of a sequence and tracks those points in consecutive projections forming trajectories. Clustering is applied to select trajectories showing an oscillating behavior similar to the breath motion. Those “breathing” trajectories are used in a 3-D reconstruction approach to recover the 3-D motion of the lung which represents the respiratory signal. Experiments were conducted on datasets exhibiting regular and irregular breathing patterns. Results showed that LIFT-based respiratory signal correlates with the diaphragm position-based signal with an average phase shift of 1.68 projections as well as with the internal marker-based signal with an average phase shift of 1.78 projections. LIFT was able to detect the respiratory signal in all projections of all datasets.
Customized Prediction of Respiratory Motion with Clustering from Multiple Patient Interaction
ACM Transactions on Intelligent Systems and Technology2013-09-01
Information processing of radiotherapy systems has become an important research area for sophisticated radiation treatment methodology. Geometrically precise delivery of radiotherapy in the thorax and upper abdomen is compromised by respiratory motion during treatment. Accurate prediction of the respiratory motion would be beneficial for improving tumor targeting. However, a wide variety of breathing patterns can make it difficult to predict the breathing motion with explicit models. We proposed a respiratory motion predictor, that is, customized prediction with multiple patient interactions using neural network (CNN). For the preprocedure of prediction for individual patient, we construct the clustering based on breathing patterns of multiple patients using the feature selection metrics that are composed of a variety of breathing features. In the intraprocedure, the proposed CNN used neural networks (NN) for a part of the prediction and the extended Kalman filter (EKF) for a part of the correction. The prediction accuracy of the proposed method was investigated with a variety of prediction time horizons using normalized root mean squared error (NRMSE) values in comparison with the alternate recurrent neural network (RNN). We have also evaluated the prediction accuracy using the marginal value that can be used as the reference value to judge how many signals lie outside the confidence level. The experimental results showed that the proposed CNN can outperform RNN with respect to the prediction accuracy with an improvement of 50%.
Irregular Breathing Classification from Multiple Patient Datasets using Neural Networks
IEEE Transactions on Information Technology in Biomedicine2012-06-01
Complicated breathing behaviors including uncertain and irregular patterns can affect the accuracy of predicting respiratory motion for precise radiation dose delivery. So far investigations on irregular breathing patterns have been limited to respiratory monitoring of only extreme inspiration and expiration. Using breathing traces acquired on a Cyberknife treatment facility, we retrospectively categorized breathing data into several classes based on the extracted feature metrics derived from breathing data of multiple patients. The novelty of this paper is that the classifier using neural networks can provide clinical merit for the statistical quantitative modeling of irregular breathing motion based on a regular ratio representing how many regular/irregular patterns exist within an observation period. We propose a new approach to detect irregular breathing patterns using neural networks, where the reconstruction error can be used to build the distribution model for each breathing class. The proposed irregular breathing classification used a regular ratio to decide whether or not the current breathing patterns were regular. The sensitivity, specificity, and receiver operating characteristiccurve of the proposed irregular breathing pattern detector was analyzed. The experimental results of 448 patients' breathing patterns validated the proposed irregular breathing classifier.
Principal Composite Kernel Feature Analysis: Data-Dependent Kernel Approach
IEEE Transactions on Knowledge Data Engineering2013-08-01
Principal composite kernel feature analysis (PC-KFA) is presented to show kernel adaptations for nonlinear features of medical image data sets (MIDS) in computer-aided diagnosis (CAD). The proposed algorithm PC-KFA has extended the existing studies on kernel feature analysis (KFA), which extracts salient features from a sample of unclassified patterns by use of a kernel method. The principal composite process for PC-KFA herein has been applied to kernel principal component analysis [34] and to our previously developed accelerated kernel feature analysis [20]. Unlike other kernel-based feature selection algorithms, PC-KFA iteratively constructs a linear subspace of a high-dimensional feature space by maximizing a variance condition for the nonlinearly transformed samples, which we call data-dependent kernel approach. The resulting kernel subspace can be first chosen by principal component analysis, and then be processed for composite kernel subspace through the efficient combination representations used for further reconstruction and classification. Numerical experiments based on several MID feature spaces of cancer CAD data have shown that PC-KFA generates efficient and an effective feature representation, and has yielded a better classification performance for the proposed composite kernel subspace using a simple pattern classifier.
A Multiple Model Approach to Tracking Head Orientation with Delta Quaternions
IEEE Transactions on Cybernetics2013-02-01
Virtual reality and augmented reality environments using helmet-mounted displays create a sense of immersion by closely coupling user head motion to display content. Delays in the presentation of visual information can destroy the sense of presence in the simulation environment when it causes a lag in the display response to user head motion. The effect of display lag can be minimized by predicting head orientation, allowing the system to have sufficient time to counteract the delay. In this paper, anew head orientation prediction technique is proposed that uses a multiple delta quaternion (DQ) extended Kalman filter to track angular head velocity and angular head acceleration. This method is independent of the device used for orientation measurement, relying on quaternion orientation as the only measurement data. A new orientation prediction algorithm is proposed that estimates future head orientation as a function of the current orientation measurement and a predicted change in orientation, using the velocity and acceleration estimates. Extensive experimentation shows that the new method improves head orientation prediction when compared to single filter DQ prediction.
Interpolation Volume Calibration: A Multisensor Calibration Technique for Electromagnetic Trackers
IEEE Transactions on Robotics2012-10-01
AC electromagnetic trackers are well suited for head tracking but are adversely affected by conductive and ferromagnetic materials. Tracking performance can be improved by mapping the tracking volume to produce coefficients that correct position and orientation (PnO) measurements caused by stationary distorting materials. The mapping process is expensive and time consuming, requiring complicated high-precision equipment to provide registration of the measurements to the source reference frame. In this study, we develop a new approach to mapping that provides registration of mapping measurements without precision equipment. Our method, i.e., the interpolation volume calibration system, uses two simple fixtures, each with multiple sensors in a rigid geometry, to determine sensor PnO in a distorted environment without mechanical measurements or other tracking technologies. We test our method in a distorted tracking environment, constructing a lookup table of the magnetic field that is used as the basis for distortion compensation. The new method compares favorably with the traditional approach providing a significant reduction in cost and effort.
Self-Reproduction for Articulated Behaviors with Dual Humanoid Robots using On-line Decision Tree Classification
Robotica2012-02-02
We have proposed a new repetition framework for vision-based behavior imitation by a sequence of multiple humanoid robots, introducing an on-line method for delimiting a time-varying context. This novel approach investigates the ability of a robot "student" to observe and imitate a behavior from a "teacher" robot; the student later changes roles to become the "teacher" for a naïve robot. For the many robots that already use video acquisition systems for their real-world tasks, this method eliminates the need for additional communication capabilities and complicated interfaces. This can reduce human intervention requirements and thus enhance the robots' practical usefulness outside the laboratory. Articulated motions are modeled in a three-layer method and registered as learned behaviors using color-based landmarks. Behaviors were identified on-line after each iteration by inducing a decision tree from the visually acquired data. Error accumulated over time, creating a context drift for behavior identification. In addition, identification and transmission of behaviors can occur between robots with differing, dynamically changing configurations. ITI, an on-line decision tree inducer in the C4.5 family, performed well for data that were similar in time and configuration to the training data but the greedily chosen attributes were not optimized for resistance to accumulating error or configuration changes. Our novel algorithm, OLDEX identified context changes on-line, as well as the amount of drift that could be tolerated before compensation was required. OLDEX can thus identify time and configuration contexts for the behavior data. This improved on previous methods, which either separated contexts off-line, or could not separate the slowly time-varying context into distinct regions at all. The results demonstrated the feasibility, usefulness, and potential of our unique idea for behavioral repetition and a propagating learning scheme.
Respiratory Motion Estimation With Hybrid Implementation of Extended Kalman Filter
IEEE Transactions on Industrial Electronics2012-11-01
The extended Kalman filter (EKF) can be used for the purpose of training nonlinear neural networks to perform desired input-output mappings. To improve the computational requirements of the EKF, Puskorius proposed the decoupled EKF (DEKF) as a practical remedy for the proper management of computational resources. This approach, however, sacrifices computational accuracy of estimates because it ignores the interactions between the estimates of mutually exclusive weights. To overcome such a limitation, therefore, we proposed hybrid implementation based on EKF (HEKF) for respiratory motion estimation, which uses the channel number for the mutually exclusive groups and the coupling technique to compensate the computational accuracy. Moreover, the authors restricted to a DEKF algorithm in which the weights connecting the inputs to a node are grouped together. If there are multiple input training sequences with respect to the time stamp, the complexity can increase by the power of the input channel number. To improve the computational complexity, we split the complicated neural network into a couple of simple neural networks to adjust separate input channels. The experimental results validated that the prediction overshoot of the proposed HEKF was improved by 62.95% in the average prediction overshoot values. The proposed HEKF showed a better performance of 52.40% improvement in the average of the prediction time horizon. We have evaluated that the proposed HEKF can outperform DEKF by comparing the prediction overshoot values, the performance of the tracking estimation value, and the normalized root-mean-squared error.
Head Orientation Prediction: Delta Quaternion versus Quaternion
IEEE Transactions on Systems, Man, and Cybernetics, Part B (Cybernetics)2009-05-01
Display lag in simulation environments with helmet-mounted displays causes a loss of immersion that degrades the value of virtual/augmented reality training simulators. Simulators use predictive tracking to compensate for display lag, preparing display updates based on the anticipated head motion. This paper proposes a new method for predicting head orientation using a delta quaternion (DQ)-based extended Kalman filter (EKF) and compares the performance to a quaternion EKF. The proposed framework operates on the change in quaternion between consecutive data frames (the DQ), which avoids the heavy computational burden of the quaternion motion equation. Head velocity is estimated from the DQ by an EKF and then used to predict future head orientation. We have tested the new framework with captured head motion data and compared it with the computationally expensive quaternion filter. Experimental results indicate that the proposed DQ method provides the accuracy of the quaternion method without the heavy computational burden.
Very Low Frequency Electromagnetic Detector With Data Acquisition
IEEE Transactions on Instrumentation and Measurement2009-01-01
Naturally occurring electromagnetic oscillating fields in the very-low-frequency (VLF) range of the spectrum, i.e., from 1 to 200 kHz, are weak and difficult to detect under normal conditions. These naturally occurring VLF electromagnetic events are observed during thunderstorms, in certain mountain winds, and during earthquakes. On the other hand, man-made VLF electromagnetic fields are stronger and have been suspected of causing negative health effects. Typical sources of these VLF emissions include television sets, video display terminals (VDTs), certain medical devices, some radio stations, and the ground-wave emergency network (GWEN) used for military communications. This paper describes the development of a triaxial ldquoVLF gaussmeter,rdquo which can be made portable. This electronic system can be used to monitor VLF electromagnetic radiation in residential and occupational environments. The ldquoVLF gaussmeterrdquo is based on a microcontroller with a built-in 10-bit A/D converter and has been designed to measure the magnetic flux density and frequency across the wide VLF bandwidth (BW). A digitized resolution of 0.2 mG is used for the 0-200-mG range, and 2-mG resolution is used for the range of 2-2000 mG. The meter has been designed to include the following features: 1) automatic or manual range selection; 2) data logging; 3) single-axis mode; 4) peak hold; 5) RS-232 communication port; and 6) analog recorder output.
Hand-Eye Calibration Applied to Viewpoint Selection for Robotic Vision
IEEE Transactions on Industrial Electronics2008-10-01
Viewpoint calibration is a method to manipulate hand-eye for generating calibration parameters for active viewpoint control and object grasping. In robot vision applications, accurate vision sensor calibration and robust vision-based robot control are essential for developing an intelligent and autonomous robotic system. This paper presents a new approach to hand-eye robotic calibration for vision-based object modeling and grasping. Our method provides a 1.0-pixel level of image registration accuracy when a standard Puma/Kawasaki robot generates an arbitrary viewpoint. To attain this accuracy, our new formalism of hand-eye calibration deals with a lens distortion model of a vision sensor. Our most distinguished approach of optimizing intrinsic parameters is to utilize a new parameter estimation algorithm using an extended Kalman filter. Most previous approaches did not even consider the optimal estimates of the intrinsic and extrinsic camera parameters, or chose one of the estimates obtained from multiple solutions, which caused a large amount of estimation error in hand-eye calibration. We demonstrate the power of this new method for: (1) generating 3-D object models using an interactive 3-D modeling editor; (2) recognizing 3-D objects using stereovision systems; and (3) grasping 3-D objects using a manipulator. Experimental results using Puma and Kawasaki robots are shown.
Video-Based Tracking and Incremental Learning Applied to Rodent Behavioral Activity under Near-Infrared Illumination
IEEE Transactions on Instrumentation and Measurement2007-12-01
This paper describes a noninvasive video tracking system for measurement of rodent behavioral activity under near-infrared (NIR) illumination, where the rodent is of a similar color to the background. This novel method allows position tracking in the dark, when rodents are generally most active, or under visible light. It also improves current video tracking methods under low-contrast conditions. We also manually extracted rodent features and classified three common behaviors (sitting, walking, and rearing) using an inductive algorithm-a decision tree (ID3). In addition, we proposed the use of a time-spatial incremental decision tree (ID5R), with which new behavior instances can be used to update the existing decision tree in an online manner. These were implemented using incremental tree induction. Open-field locomotor activity was investigated under ldquovisiblerdquo ( ), 880- and 940-nm wavelengths of NIR, as well as a ldquodarkrdquo condition consisting of a very small level of NIR illumination. A widely used NIR crossbeam-based tracking system (Activity Monitor, MED Associates, Inc., Georgia, VT) was used to record simultaneous position data for validation of the video tracking system. The classification accuracy for the set of new test data was 81.3%.
An Interactive Framework for Acquiring Vision Models of 3D Objects from 2D images
IEEE Transactions on Systems, Man, and Cybernetics, Part B (Cybernetics)2004-02-01
This paper presents a human-computer interaction (HCI) framework for building vision models of three-dimensional (3-D) objects from their two-dimensional (2-D) images. Our framework is based on two guiding principles of HCI: 1) provide the human with as much visual assistance as possible to help the human make a correct input; and 2) verify each input provided by the human for its consistency with the inputs previously provided. For example, when stereo correspondence information is elicited from a human, his/her job is facilitated by superimposing epipolar lines on the images. Although that reduces the possibility of error in the human marked correspondences, such errors are not entirely eliminated because there can be multiple candidate points close together for complex objects. For another example, when pose-to-pose correspondence is sought from a human, his/her job is made easier by allowing the human to rotate the partial model constructed in the previous pose in relation to the partial model for the current pose. While this facility reduces the incidence of human-supplied pose-to-pose correspondence errors, such errors cannot be eliminated entirely because of confusion created when multiple candidate features exist close together. Each input provided by the human is therefore checked against the previous inputs by invoking situation-specific constraints. Different types of constraints (and different human-computer interaction protocols) are needed for the extraction of polygonal features and for the extraction of curved features. We will show results on both polygonal objects and object containing curved features.
Respiratory motion prediction and perspective correction for free-breathing arterial spin labeled perfusion MRI of the kidney
Medical Physics2017-01-01
Purpose
Respiratory motion prediction using an artificial neural network (ANN) was integrated with pseudocontinuous arterial spin labeling (pCASL) MRI to allow free-breathing perfusion measurements in the kidney. In this study, we evaluated the performance of the ANN to accurately predict the location of the kidneys during image acquisition.
Methods
A pencil-beam navigator was integrated with a pCASL sequence to measure lung/diaphragm motion during ANN training and the pCASL transit delay. The ANN algorithm ran concurrently in the background to predict organ location during the 0.7 s 15-slice acquisition based on the navigator data. The predictions were supplied to the pulse sequence to prospectively adjust the axial slice acquisition to match the predicted organ location. Additional navigators were acquired immediately after the multislice acquisition to assess the performance and accuracy of the ANN. The technique was tested in 8 healthy volunteers.
Results
The root mean square error (RMSE) and mean absolute error (MAE) for the 8 volunteers were 1.91 ± 0.17 mm and 1.43 ± 0.17 mm, respectively, for the ANN. The RMSE increased with transit delay. The MAE typically increased from the first to last prediction in the image acquisition. The overshoot was 23.58% ± 3.05% using the target prediction accuracy of ± 1 mm.
Conclusion
Respiratory motion prediction with prospective motion correction was successfully demonstrated for free-breathing perfusion MRI of the kidney. The method serves as an alternative to multiple breathholds and requires minimal effort on the part of the patient.
Multicolumn RBF Network
IEEE Transactions on Neural Networks and Learning Systems2017-01-01
This study proposes the multi-column RBF network (MCRN) as a method to improve the accuracy and speed of a traditional radial basis function network (RBFN). The RBFN, as a fully connected artificial neural network (ANN), suffers from costly kernel inner-product calculations due to the use of many instances as the centers of hidden units. This issue is not critical for small datasets, as adding more hidden units will not burden the computation time. However, for larger datasets, the RBFN requires many hidden units with several kernel computations to generalize the problem. The MCRN mechanism is constructed based on dividing a dataset into smaller subsets using the k-d tree algorithm. N resultant subsets are considered as separate training datasets to train N individual RBFNs. Those small RBFNs are stacked in parallel and bulged into the MCRN structure during testing. The MCRN is considered as a well-developed and easy-to-use parallel structure because each individual ANN has been trained on its own subsets and is completely separate from the other ANNs. This parallelized structure reduces the testing time compared to that of a single but larger RBFN, which cannot easily be parallelized due to its fully connected structure. Small informative subsets provide the MCRN with a regional experience to specify the problem instead of generalizing it. The MCRN has been tested on many benchmark datasets and has shown better accuracy and great improvements in training and testing times compared to a single RBFN. The MCRN also shows good results compared to those of some machine learning techniques, such as the support vector machine (SVM) and k-nearest neighbors (KNN).
A Novel Method of Cone Beam CT Projection Binning base on Image Registration
IEEE Transactions on Medical ImagingS. Park, S. Kim, B. Yi, G. Hugo, H. Gach, and Y. Motai
2017-03-01
Accurate sorting of beam projections is important in four-dimensional Cone Beam Computed Tomography (4D CBCT) to improve the quality of the reconstructed 4D CBCT image by removing motion-induced artifacts. We propose Image Registration-based Projection Binning (IRPB), a novel marker-less binning method for 4D CBCT projections, which combines Intensity-based Feature Point Detection (IFPD) and Trajectory Tracking using Random sample consensus (TTR). IRPB extracts breathing motion and phases by analyzing tissue feature point trajectories. We conducted experiments with two phantom and six patient datasets including both regular and irregular respiration. In experiments, we compared the performance of the proposed IRPB, Amsterdam Shroud method (AS), Fourier Transform-based method (FT), and Local Intensity Feature Tracking (LIFT) method. The results showed that the average absolute phase shift of IRPB was 3.74 projections and 0.48 projections less than that of FT and LIFT, respectively. AS lost the most breathing cycles in the respiration extraction for the five patient datasets, so we could not compare the average absolute phase shift between IRPB and AS. Based on the Peak Signal-to-Noise Ratio (PSNR) of the reconstructed 4D CBCT images, IRPB had 5.08 dB, 1.05 dB, and 2.90 dB larger PSNR than AS, FT, and LIFT, respectively. The average Structure SIMilarity Index (SSIM) of the 4D CBCT image reconstructed by IRPB, AS, and LIFT were 0.87, 0.74, 0.84, and 0.70, respectively. These results demonstrated that IRPB has superior performance to the other standard methods.
Acoustic Fault Detection Technique for High Power Insulators
IEEE Transactions on Industrial ElectronicsK. Park, Y. Motai*, and J. Yoon
2017-03-01
Insulators are important equipment used to electrically isolate and mechanically hold wires in high-voltage power transmission systems. Faults caused by the deterioration of the insulators induce very serious problems to the power transmission line. Techniques were introduced for the acoustic detection of insulator faults by acoustic radiation noises. Radiation noises were measured from normal state insulators and fault state insulators in an anechoic chamber. The insulators used were two porcelain insulators, a cut out switch, two line posters, and a lightning arrester. A new acoustic technique determines the direction of the insulator faults using source localization with 3D microphone arrays. The advantage is to classify the fault state insulators without human inspection by considering the amount of total noises and 120Hz harmonic components. The fault detection was determined by neural network to diagnose the state automatically. The proposed technique was evaluated by distinct, real datasets and the efficacy was validated. The noise source was detected with 100.0% accuracy and the classification ratio achieved 96.7% for three typical conditions.
Human Behavior-based Target Tracking with an Omni-directional Thermal Camera
IEEE Transactions on Cognitive and Developmental SystemsE. Benrli, Y. Motai* and J. Rogers
2017-06-01
We investigate Human Behavior-based Target Tracking from Omni-directional (O-D) thermal images for intelligent perception in unmanned systems. Current target tracking approaches are primarily focused on perspective visual and infrared band, as well as O-D visual band tracking. The target tracking from O-D images and the use of O-D thermal vision have not been adequately addressed. Thermal O-D images provide a number of advantages over other passive sensor modalities such as illumination invariance, wide field-of-view, ease of identifying heat-emitting objects, and long term tracking without interruption. Unfortunately, thermal O-D sensors have not yet been widely used due to the following disadvantages: low resolution, low frame rates, high cost, sensor noise, and an increase in tracking time. This paper outlines a spectrum of approaches which mitigate these disadvantages to enable an O-D thermal IR camera equipped with a mobile robot to track a human in a variety of environments and conditions. The CMKF (Curve Matched Kalman Filter) is used for tracking a human target based on the behavioral movement of the human and MAP (Maximum A Posteriori) based estimation is extended for the human tracking as long term which provides a faster prediction. The benefits to using our MAP based method are decreasing the prediction time of a target's position and increasing the accuracy of prediction of the next target position based on the target’s previous behavior while increasing the tracking view and lighting conditions via the view from O-D IR camera.
Dynamic 3D Reconstruction of Human Targets via an Omni-directional Infrared Sensor
IEEE Sensors JournalE. Benrli, J. Rahlf, and Y. Motai*
2018-08-01
We explore the dynamic 3-D reconstruction (D3DR) of the target view in real-time images from the omni-directional (O-D) thermal sensor for intelligent perception of robotic systems. Recent O-D 3-D reconstruction methodologies are mainly focused on O-D visible-band vision for localization, mapping, calibration, and tracking, but there is no significant research for thermal O-D. The 3-D reconstruction from O-D images and the use of O-D thermal vision have not been sufficiently addressed. The thermal O-D images do not provide sharp-edge boundaries as in color vision cameras due to texture and mirror distortion. In order to fully address O-D thermal 3-D reconstruction, we proposed the D3DR method that dynamically detects the target region and densely reconstructs the detected target region to solve the non-sharp-edge boundaries' issue. We analyzed several different imaging positions, different baseline distances, and target distances with respect to the robot position for the best coverage of the target view with a minimum reconstruction error. We also look at the optimum number of observations for reconstruction using an optimization to find the compromise between accuracy, methodology, and number of observations. The benefits of this method are the accurate distance of the target from the camera, high accuracy, and low computation time of 3-D reconstruction.
Vegetation Segmentation for Sensor Fusion of Omnidirectional Far-infrared and Visual Stream
IEEE Journal of Selected Topics in Applied Earth Observations and Remote SensingD. Stone, G. Shah, Y. Motai*, and A. Aved
2018-08-01
In the context of vegetation detection, the fusion of omnidirectional (O-D) infrared (IR) and color vision sensors may increase the level of vegetation perception for unmanned robotic platforms. Current approaches are primarily focused on O-D color vision for localization, mapping, and tracking. A literature search found no significant research in our area of interest. The fusion of O-D IR and O-D color vision sensors for the extraction of feature material type has not been adequately addressed. We will look at augmenting indices-based spectral decomposition with IR region-based spectral decomposition to address the number of false detects inherent in indices-based spectral decomposition alone. Our work shows that the fusion of the normalized difference vegetation index (NDVI) from the O-D color camera fused with the IR thresholded signature region associated with the vegetation region minimizes the number of false detects seen with NDVI alone. The contribution of this paper is the demonstration of a new technique, thresholded region fusion technique for the fusion of O-D IR and O-D color. We also look at the Kinect vision sensor fused with the O-D IR camera. Our experimental validation demonstrates a 64% reduction in false detects in our method compared to classical indices-based detection.
Detecting Infrared Maritime Targets Overwhelmed in Sun Glitters by Anti-Jitter Spatiotemporal Saliency
IEEE Transaction on Geoscience and Remote SensingB. Wang, Y. Motai*, L. Dong, and W. Xu
2018-08-01
When detecting infrared maritime targets on sunny days, strong sun glitters can lower the detection accuracy tremendously. To this problem, we proposed a robust antijitter spatiotemporal saliency generation with parallel binarization (ASSGPB) method. Its main contribution is to facilitate to improve the infrared maritime target detection accuracy in this situation. The ASSGPB algorithm exploits the target's spatial saliency and temporal consistency to separate real targets from clutter areas. The ASSGPB first corrects image intensity distribution with a central inhibition difference of Gaussian filter. Then, a self-defined spatiotemporal saliency map (STSM) generator is used to generate an STSM in five consecutive frames while compensating interframe jitters by a joint block matching. Finally, a parallel binarization method is adopted to segment real targets in STSM while keeping full target areas. To evaluate the performance of ASSGPB, we captured eight different image sequences (20,420 frames in total) that were significantly contaminated by strong sun glitters. The ASSGPB realized 100% detection rate and 0.45% false alarm rate in these data sets, greatly outperforming four state-of-the-art algorithms. Thus, a great applicability of ASSGPB has been verified through our experiments.
Direct Spherical Calibration of Omnidirectional Far Infrared Camera System
IEEE Sensors JournalD. Stone, G. Shah, and Y. Motai*
2018-08-01
We investigates the calibration of omnidirectional infrared (IR) camera for intelligent perception applications. Current omnidirectional camera approaches are primarily focused on omnidirectional color vision applications. The low resolution omnidirectional (O-D) IR image edge boundaries are not as sharp as with color vision cameras, and as a result, the standard calibration methods were harder to use and less accurate with the low definition of the omnidirectional IR camera. In order to more fully address omnidirectional IR camera calibration, we propose a new calibration grid center coordinates control point discovery methodology and a Direct Spherical Calibration (DSC) approach for a more robust and accurate method of calibration. DSC will address the limitations of the existing methods by using the spherical coordinates of the centroid of the calibration board to directly triangulate the location of the camera center and iteratively solve for the camera parameters. We compare DSC to three Baseline visual calibration methodologies and augment them with additional output of the spherical results for comparison. We also look at the optimum number of calibration boards using an evolutionary algorithm and Pareto optimization to find the best method and combination of accuracy, methodology and number of calibration boards. The benefits of DSC are more efficient calibration board geometry selection, and better accuracy than the three Baseline visual calibration methodologies.
Thermal Multisensor Fusion for Collaborative Robotics
IEEE Transactions on Industrial InformaticsE. Benrli, R. L. Spidalieri, and Y. Motai*
2018-08-01
Collaborative robotic configurations for monitoring and tracking human targets have attracted interest for the 4th industrial revolution. The fusion of different types of sensors embedded in collaborative robotic systems achieves high quality information and contributes to significantly improve robotic perception. However, current methods have not deeply explored the capabilities of thermal multisensory configurations in human-oriented tasks. We propose Thermal Multisensor Fusion (TMF) for collaborative robots to overcome the limitations of stand-alone robots. Thermal vision helps to utilize the heat signature of the human body for human oriented tracking. An omni-directional (O-D) infrared (IR) sensor provides a wide field of view to detect human targets and Stereo IR helps determine the distance of the human target in the oriented direction. The fusion of O-D IR and Stereo IR also creates a multi-sensor stereo for an additional determination of distance to the target. The fusion of thermal and O-D sensors bring their limited prediction accuracy with their advantages. The Maximum A Posteriori (MAP) method is used to predict the distance of the target with high accuracy by using the distance results of TMF stereo from multiple platforms according to the reliability of the sensors rather than its usage of visible-band-based tracking methods. The proposed method tracks the distance calculation of each sensor instead of target trajectory tracking as in visible-band methods. We proved that TMF increases the perception of robots by offering a wide field of view and provides precise target localization for collaborative robots.
Elastographic Tomosynthesis from X-ray Strain Imaging of Breast Cancer
IEEE Journal of Translational Engineering in Health and MedicineC. Sutphin, E. Olson, Y. Motai*, S. J. Lee, J. G. Kim, and K. Takabe
2019-08-19
Noncancerous breast tissue and cancerous breast tissue have different elastic properties. In particular, cancerous breast tumors are stiff when compared to the noncancerous surrounding tissue. This difference in elasticity can be used as a means for detection through the method of elastographic tomosynthesis by means of physical modulation. This paper deals with a method to visualize elasticity of soft tissues, particularly breast tissues, via x-ray tomosynthesis. X-ray tomosynthesis is now used to visualize breast tissues with better resolution than the conventional single-shot mammography. The advantage of X-ray tomosynthesis over X-ray CT is that fewer projections are needed than CT to perform the reconstruction, thus radiation exposure and cost are both reduced. Two phantoms were used for the testing of this method, a physical phantom and an in silico phantom. The standard root mean square error in the tomosynthesis for the physical phantom was 2.093 and the error in the in silico phantom was negligible. The elastographs were created through the use of displacement and strain graphing. A Gaussian Mixture Model with an expectation-maximization clustering algorithm was applied in three dimensions with an error of 16.667%. The results of this paper have been substantial when using phantom data. There are no equivalent comparisons yet in 3D x-ray elastographic tomosynthesis. Tomosynthesis with and without physical modulation in the 3D elastograph can identify feature groupings used for biopsy. The studies have potential to be applied to human test data used as a guide for biopsy to improve accuracy of diagnosis results. Further research on this topic could prove to yield new techniques for human patient diagnosis purposes.
Electric Load Forecasting Model Using a Multicolumn Deep Neural Networks
IEEE Transactions on Industrial ElectronicsA. Hoori, A.A. Kazzaz, R. Khimani, Y. Motai*, and A. Aved
2019-09-12
In this article, a new approach to short-term load forecasting is proposed using a multicolumn radial basis function neural network (MCRN). The advantage of this new approach over similar models in speed and accuracy is also discussed, especially in regards to renewable generation forecasting. Because weather and seasonal effects have a direct impact not only on load demand but also on renewable energy production, it follows that as the penetration rate of renewable DG increases, the grid will become even more sensitive to weather impacts in the long term. In our approach, we use a k-d tree algorithm to split our feature-rich dataset into dense specialized subsets. These subsets are then trained in parallel as multiple artificial neural networks using a modified error correction algorithm to form the MCRN. This approach reduces the number of hidden neurons, increases the speed of convergence, and improves generalization over similar alternative forecasting methods.
Visual Perception for Multiple Human-Robot Interaction from Motion Behavior
IEEE Systems JournalE. Benrli, Y. Motai*, and J. Rogers
2019-12-27
Visual perception is an important component for human–robot interaction processes in robotic systems. Interaction between humans and robots depends on the reliability of the robotic vision systems. The variation of camera sensors and the capability of these sensors to detect many types of sensory inputs improve the visual perception. The analysis of activities, motions, skills, and behaviors of humans and robots have been addressed by utilizing the heat signatures of the human body. The human motion behavior is analyzed by body movement kinematics, and the trajectory of the target is used to identify the objects and the human target in the omnidirectional (O-D) thermal images. The process of human target identification and gesture recognition by traditional sensors have problem for multitarget scenarios since these sensors may not keep all targets in their narrow field of view (FOV) at the same time. O-D thermal view increases the robots’ line-of-sights and ability to obtain better perception in the absence of light. The human target is informed of its position, surrounding objects and any other human targets in its proximity so that humans with limited vision or vision disability can be assisted to improve their ability in their environment. The proposed method helps to identify the human targets in a wide FOV and light independent conditions to assist the human target and improve the human–robot and robot–robot interactions. The experimental results show that the identification of the human targets is achieved with a high accuracy.
Robust Detection of Infrared Maritime Targets for Autonomous Navigation
IEEE Transactions on Intelligent VehiclesB.Wang, E. Benli, Y.Motai*, L.Dong, and W.Xu
2020-05-06
This paper addresses a problem on infrared maritime target detection robustly in various situations. Its main contribution is to improve the infrared maritime target detection accuracy in different backgrounds, for various targets, using multiple infrared wave bands. The accuracy and the computational time of traditional infrared maritime searching systems are improved by our proposed Local Peak Singularity Measurement (LPSM)-Based Image Enhancement and Grayscale Distribution Curve Shift Binarization (GDCSB)-Based Target Segmentation. The first part uses LPSM to quantize the local singularity of each peak. Additionally, an enhancement map (EM) is generated based on the quantitative local singularity. After multiplying the original image by the EM, targets can be enhanced and the background will be suppressed. The second part of GDCSB-Based Target Segmentation calculates the desired threshold by cyclic shift of the grayscale distribution curve (GDC) of the enhanced image. After binarizing the enhanced image, real targets can be segmented from the image background. To verify the proposed algorithm, experiments based on 13,625 infrared maritime images and five comparison algorithms were conducted. Results show that the proposed algorithm has solid performance in strong and weak background clutters, different wave bands, different maritime targets, etc.
DeepFuseNet of Omnidirectional Far-infrared and Visual Stream for Vegetation Detection
IEEE Transaction on Geoscience and Remote SensingThis article investigates the application of deep learning (DL) to the fusion of omnidirectional (O-D) infrared (IR) sensors and O-D visual sensors to improve the intelligent perception of autonomous robotic systems. Recent techniques primarily focus on O-D and conventional visual sensors for applications in localization, mapping, and tracking. The robotic vision systems have not sufficiently utilized the combination of O-D IR and O-D visual sensors, coupled with DL, for the extraction of vegetation material. We will be showing the contradiction between current approaches and our deep vegetation learning sensor fusion. This article introduces two architectures: 1) the application of two autoencoders feeding into a four-layer convolutional neural network (CNN) and 2) two deep CNN feature extractors feeding a deep CNN fusion network (DeepFuseNet) for the fusion of O-D IR and O-D visual sensors to better address the number of false detects inherent in indices-based spectral decomposition. We compare our DL results to our previous work with normalized difference vegetation index (NDVI) and IR region-based spectral fusion, and to traditional machine learning approaches. This work proves that the fusion of the O-D IR and O-D visual streams utilizing our DeepFuseNet DL approach outperforms both the previous NVDI fused with far-IR region segmentation and traditional machine learning approaches. Experimental results of our method validate a 92% reduction in false detects compared to traditional indices-based detection. This article contributes a novel method for the fusion of O-D visual and O-D IR sensors using two CNN feature extractors feeding into a deep CNN (DeepFuseNet).
Autoencoder-Inspired Convolutional Network-Based Super-Resolution Method in MRI
IEEE Journal of Translational Engineering in Health and MedicineObjective: To introduce an MRI in-plane resolution enhancement method that estimates High-Resolution (HR) MRIs from Low-Resolution (LR) MRIs. Method & Materials: Previous CNN-based MRI super-resolution methods cause loss of input image information due to the pooling layer. An Autoencoder-inspired Convolutional Network-based Super-resolution (ACNS) method was developed with the deconvolution layer that extrapolates the missing spatial information by the convolutional neural network-based nonlinear mapping between LR and HR features of MRI. Simulation experiments were conducted with virtual phantom images and thoracic MRIs from four volunteers. The Peak Signal-to-Noise Ratio (PSNR), Structure SIMilarity index (SSIM), Information Fidelity Criterion (IFC), and computational time were compared among: ACNS; Super-Resolution Convolutional Neural Network (SRCNN); Fast Super-Resolution Convolutional Neural Network (FSRCNN); Deeply-Recursive Convolutional Network (DRCN). Results: ACNS achieved comparable PSNR, SSIM, and IFC results to SRCNN, FSRCNN, and DRCN. However, the average computation speed of ACNS was 6, 4, and 35 times faster than SRCNN, FSRCNN, and DRCN, respectively under the computer setup used with the actual average computation time of 0.15 s per 100×100 pixels. Conclusion: The result of this study implies the potential application of ACNS to real-time resolution enhancement of 4D MRI in MRI guided radiation therapy.